勇哥买了一台10核心20线程的32G内存的服务器, 一时好奇, 想试试并发运算的情况下, cpu的表现如何 .
字符串相关度计算是文本处理和数据挖掘中一个不可或缺的方法,例如论文查重等。Levenshtein Distance可以用来比较两个字符串的相似度,即两个字符串之间的“距离”。这个“距离”其实就是从源字符串变换到目标字符串需要进行的删除、插入和替换的次数。
有不少该算法的改进版本,可大幅度提升效率,不过这不是本文的目的。我们的目的就是看看该算法在不同的机器下到底能跑多快,再看并行优化后又能跑多快。该算法基本只对CPU性能敏感,很适合做测试。
我们随机生成1000个1000长度的字符串,并比较字符串相关度。示例工程可在文末找到,由于需要并行计算,需要VS2010,.NET4.0支持。
代码如下:
namespace SpeedTest { using System; using System.Diagnostics; using System.Text; using System.Threading.Tasks; internal class Program { #region Methods private static void Main(string[] args) { var watch =new Stopwatch(); const long count = 1000; const int length = 1000; string comparestring = StringDistance.GenerateRandomString(length); var strlist = new string[count]; var steps = new int[count]; // prepare string[] for comparison Parallel.For(0, count, i => strlist[i] = StringDistance.GenerateRandomString(length)); Console.WriteLine("已经生成了" + count + "个长度为" + length + "的字符串"); watch.Start(); for (int i = 0; i < count; i++) { steps[i] = StringDistance.LevenshteinDistance(comparestring, strlist[i]); } watch.Stop(); Console.WriteLine("完成非并行计算,耗时(ms)"+watch.ElapsedMilliseconds); Console.WriteLine("性能比" + 100000d/watch.ElapsedMilliseconds); watch.Reset(); watch.Start(); Parallel.For( 0, count, delegate(long i) { steps[i] = StringDistance.LevenshteinDistance(comparestring, strlist[i]); }); watch.Stop(); Console.WriteLine("完成并行计算,耗时(ms)" + watch.ElapsedMilliseconds); Console.WriteLine("性能比" + 100000d / watch.ElapsedMilliseconds); Console.ReadKey(); Console.ReadKey(); } #endregion } internal class StringDistance { #region Public Methods public static string GenerateRandomString(int length) { var r = new Random((int)DateTime.Now.Ticks); var sb = new StringBuilder(length); for (int i = 0; i < length; i++) { int c = r.Next(97, 123); sb.Append(Char.ConvertFromUtf32(c)); } return sb.ToString(); } public static int LevenshteinDistance(string str1, string str2) { var scratchDistanceMatrix = new int[str1.Length + 1,str2.Length + 1]; // distance matrix contains one extra row and column for the seed values for (int i = 0; i <= str1.Length; i++) { scratchDistanceMatrix[i, 0] = i; } for (int j = 0; j <= str2.Length; j++) { scratchDistanceMatrix[0, j] = j; } for (int i = 1; i <= str1.Length; i++) { int str1Index = i - 1; for (int j = 1; j <= str2.Length; j++) { int str2Index = j - 1; int cost = (str1[str1Index] == str2[str2Index]) ? 0 : 1; int deletion = (i == 0) ? 1 : scratchDistanceMatrix[i - 1, j] + 1; int insertion = (j == 0) ? 1 : scratchDistanceMatrix[i, j - 1] + 1; int substitution = (i == 0 || j == 0) ? cost : scratchDistanceMatrix[i - 1, j - 1] + cost; scratchDistanceMatrix[i, j] = Math.Min(Math.Min(deletion, insertion), substitution); // Check for Transposition if (i > 1 && j > 1 && (str1[str1Index] == str2[str2Index - 1]) && (str1[str1Index - 1] == str2[str2Index])) { scratchDistanceMatrix[i, j] = Math.Min( scratchDistanceMatrix[i, j], scratchDistanceMatrix[i - 2, j - 2] + cost); } } } // Levenshtein distance is the bottom right element return scratchDistanceMatrix[str1.Length, str2.Length]; } #endregion } }
下面是debug模式的执行程序运行速度。
下面是release版本的执行速度。
下图是并行计算对CPU的占用,可以明显看到打出一波80%以上cpu的占用时间。
其它平坦的部分是非并行计算时候的cpu占用时间.
下图是不同CPU串行速度的对比图:
我们看到基本上同平台,同架构的CPU间,频率是王道。而当前I3新酷睿都可以干掉当年桌面的老酷睿经典,不得不说技术发展迅速。而服务器核心虽然核心多,甚至是双CPU,但频率一般,因此在这种普通应用下甚至抗不过桌面的I5 2500K。 能多核优化的程序还是占少数,大家能超就超一点吧,超频带来的性能提升真心不是一点点。
下图是并行下速度对比图:
并行计算下,核的数量是王道!在非服务器核心上,加速比几乎就是CPU核心数。四核的速度就是比双核的快。在八核的顶级服务器CPU上,它的速度几乎是AMD QL-62的13倍!
总体来说,单核性能频率和架构决定一切,多核性能上核心数决定了加速比。 桌面级应用完全没必要买服务器的多核心(E3 1230这类除外)。大部分程序最多只对四核CPU做过优化。更多核心甚至双CPU会造成各种不兼容性问题,连游戏都打不了(嘿嘿),编程都卡。
现在觉得,I5 2500K还是要比E3 1230性价比高很多,超频超出来的性能不是盖的。别担心寿命,它只会在需要的时候睿频到更高的频率,平时都是乖乖的二点几吉赫兹。而且非常简单!Enjoy it!
程序并行的优势还是非常明显的,.NET的并行库可比Intel并行库方便多了(虽然效率不能比),但它可以很好的帮助你挖掘多核CPU的潜力。大家可以多学习一下.NET并行库。
勇哥测试的电脑cpu是E5-2650 V3, 十核心20线程的服务器CPU。比起本文的E5 2690八核心, 其结果比较近似.
---------------------
作者:hackpig
来源:www.skcircle.com
版权声明:本文为博主原创文章,转载请附上博文链接!
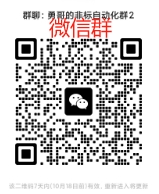
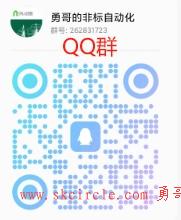